Novel Deep Learning for Knee Abnormalities
This is a news story, published by ScienceDaily, that relates primarily to the Hong Kong University of Science and Technology news.
biology news
For more biology news, you can click here:
more biology newsScienceDaily news
For more news from ScienceDaily, you can click here:
more news from ScienceDailyAbout the Otherweb
Otherweb, Inc is a public benefit corporation, dedicated to improving the quality of news people consume. We are non-partisan, junk-free, and ad-free. We use artificial intelligence (AI) to remove junk from your news feed, and allow you to select the best science news, business news, entertainment news, and much more. If you like biology news, you might also like this article about
knee pathology. We are dedicated to bringing you the highest-quality news, junk-free and ad-free, about your favorite topics. Please come every day to read the latest Various knee abnormalities news, novel deep learning model news, biology news, and other high-quality news about any topic that interests you. We are working hard to create the best news aggregator on the web, and to put you in control of your news feed - whether you choose to read the latest news through our website, our news app, or our daily newsletter - all free!
knee abnormalitiesScienceDaily
•Diagnosing knee abnormalities like an experienced radiologist: A novel deep learning model
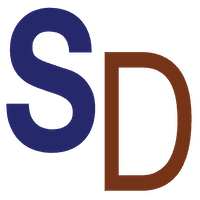
82% Informative
Multi-sequence knee magnetic resonance imaging (MRI) is an advanced non-invasive diagnostic method for knee pathology.
MRI interpretation is highly time-consuming and heavily dependent on expertise.
A research team from the Hong Kong University of Science and Technology (HKUST) has introduced a novel deep learning model which can assist with classifying 12 common types of knee abnormalities.
VR Score
91
Informative language
98
Neutral language
56
Article tone
formal
Language
English
Language complexity
82
Offensive language
not offensive
Hate speech
not hateful
Attention-grabbing headline
not detected
Known propaganda techniques
not detected
Time-value
long-living
External references
no external sources
Source diversity
no sources
Affiliate links
no affiliate links